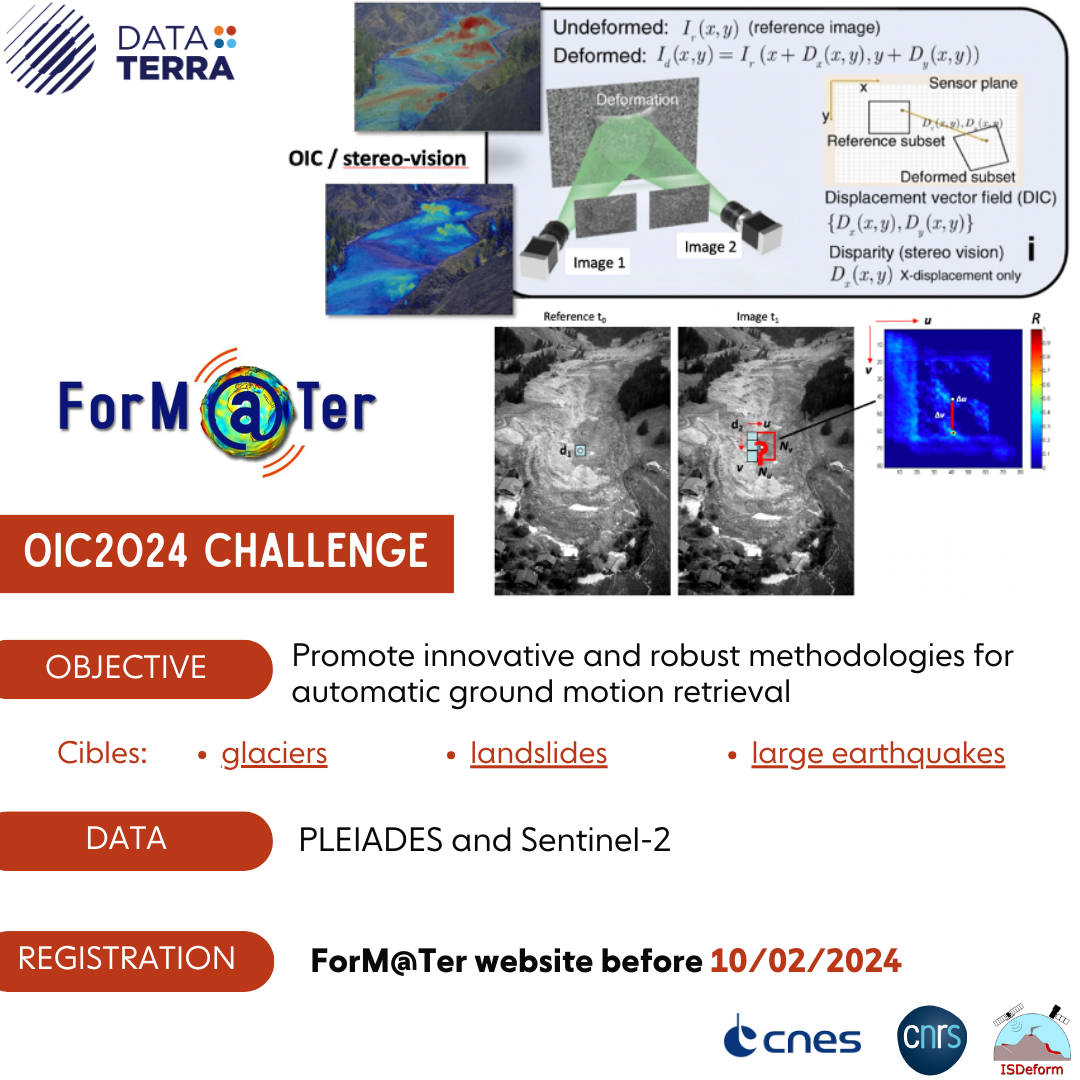
The ForM@Ter OIC2024 Challenge, organized by ForM@Ter, the Solid Earth data hub of the Research Infrastructure Data Terra, with the support of CNRS (Centre National de la Recherche Scientifique), CNES (French Space Agency), and in close collaboration with ISDeform (National Service on Earth Terrain motion monitoring from Space), aims to promote research in the use of satellite offset tracking techniques applied to optical imagery (e.g. OIC – Optical Image Correlation) for the detection and quantification of displacements and velocity of various geological objects (glaciers, landslides, large earthquakes).
Terrain motion is a variable used in various fields of Earth sciences for understanding the physical mechanisms controlling fault, landslide, glacier or volcano activity and can be a key parameter for Disaster Risk Management (DRM) applications.
Among the numerous techniques measuring the ground motion (e.g. GNSS, levelling, etc.), space-borne sensing of the Earth surface provides a global coverage and allows measurement over extended areas. Numerous satellite constellations, in particular optical satellite imagery of high spatial resolution are now publicly available such as the Copernicus Sentinels (Copernicus, > 2015), the NASA long archive of the Landsat missions (since 1970), and very-high resolution satellite imagery (e.g. Maxar Worldview/RapidEye, ADS Spot/Pléiades, Planetscope) is becoming more and more accessible. These datasets represent an interesting source of information for ground deformation monitoring because of their high frequency of revisit, their spatial resolution and, for some of them (Sentinels, Landsat), their open-access data policy.
OIC (i.e. pixel offset tracking) can measure surface motion by determining the offset between two satellite acquisitions. In the case of optical satellite with off-nadir look angles, the technique is sensitive to displacement in the horizontal plane. Depending on the ground sampling distance (i.e. satellite image spatial resolution), the technique is sensitive to large displacement magnitudes from tens of centimeters to meters.
To improve the measurement resolution and accuracy, several matching algorithms (e.g. subpixel OIC refinement methods) do exist and among them SGM/Semi-Global Matching (Hirschmuller et al., 2008: 10.1109/TPAMI.2007.1166), MGM / More Global Matching (Faciolo et al., 2015: 10.5244/C.29.90), phase frequency correlation (Van Puymbroeck et al., 2000: 10.1364/AO.39.003486) and optical flow (Lucas & Kanade, 1981: 10.5555/1623264.1623280). Recently, deep learning (Pang et al., 2018: 10.1109/ICCVW.2017.108) has also been applied to image matching in order to achieve improved performance compared with the traditional sub-pixel refinement methods.
Many open source libraries implementing these approaches are accessible, and among them (the provided list in non-exhaustive), MicMac, ASP, Cosi-Corr, QPEC/MEdicis, S2P, CARS, GeFolKi and several Deep Learning CNN based models (for instance: https://entrepot.recherche.data.gouv.fr/dataset.xhtml?persistentId=doi:10.57745/UOGRPY).
All these methods and algorithms are sensitive to the image acquisition geometry, the radiometry/texture of the images, the type of deformation (rigid, non-rigid), the amplitude of deformation and the quality of the co-registration/alignment of the image pairs.
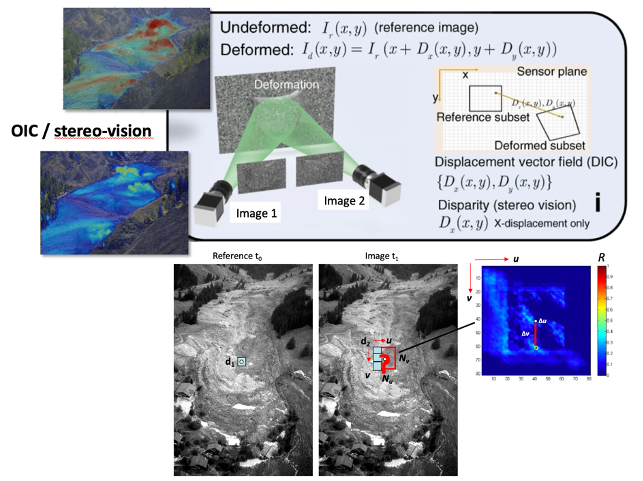
The aim of the ForM@Ter OIC2024 Challenge is to promote innovative and robust methodologies for automatic ground motion retrieval from known Sentinel-2 and Pléiades datasets for three categories of deforming objects : glaciers, landslides, earthquakes, and to provide objective and fair comparisons among the different methods and/or pipelines.
There is no ranking sensu-stricto. The participants will be invited to participate to a workshop to be hold in Toulouse (France) in June 2024 in which all results will be presented and discussed, and a collaborative paper will be written after the workshop (with a target submission by November 2024).
The challenge is organized in four phases
Phase 1 (January 2024): Interested participants need to register on-line on the ForM@Ter OIC2024 Challenge form on this page, tab “Registration”, by 10th February 2024.
Phase 2 (February – May 2024): Participants are provided with all the input satellite image datasets, background information (DSM, landcover) and the expected outputs specifications, in order to start their processing. Participants will submit their ground motion displacement maps, and relevant quality indicators, to a dedicated repository. The processing methodology will need to be described using a template that will be provided.
Phase 3 (May-June 2024): The organizers of the challenge will compare and evaluate all the results and propose relevant quality comparison metrics. The products being evaluated are:
- the “raw” versions of the OIC displacement products (to gain information on the sensitivity and accuracy of the diverse approaches/methodologies),
- the estimation of the final/best displacement/velocity products including post-processing according to reference measurements (e.g. GNSS, LiDAR, field measurements)
Phase 4 (end June 2024):
All participants will be invited to the ForM@Ter OIC2024 Challenge workshop, to be organized end June 2024 in Toulouse, and will be able to present and discuss their work.
Data Description
The ForM@Ter OIC2024 Challenge dataset consists of a series of Sentinel-2 and Pléiades orthorectified satellite imagery acquired over three areas:
- The Mont-Blanc Massif / Argentière glacier (Sentinel-2 archive 2016-2022 / Pléiades imagery)
- South France / La Valette landslide (Sentinel-2 archive 2016-2022 / Pléiades imagery)
- California / Ridgecrest earthquake (Sentinel-2 acquisitions of June-July 2019 / Pléiades imagery)
Additional base layers (DSM, landcover) and the image metadata will be provided. The dataset, for licensing issues, will be provided only to the registered participants, at the start of Challenge Phase 2.
The participants are encouraged to process the three datasets to offer the opportunity of a transdisciplinary inter-comparison among the glaciology, the landslide and earthquake research communities.
Challenge Organizers
Centre National de la Recherche Scientifique / ForM@Ter: Floriane Provost (EOST), Laurane Charrier (IGE), Bastien Wirtz (EOST), Arthur Delorme (IPGP), Pascal Lacroix (ISTerre), Jean-Philippe Malet (EOST)
Centre National des Etudes Spatiales / ForM@Ter : Claude Boniface (CNES)